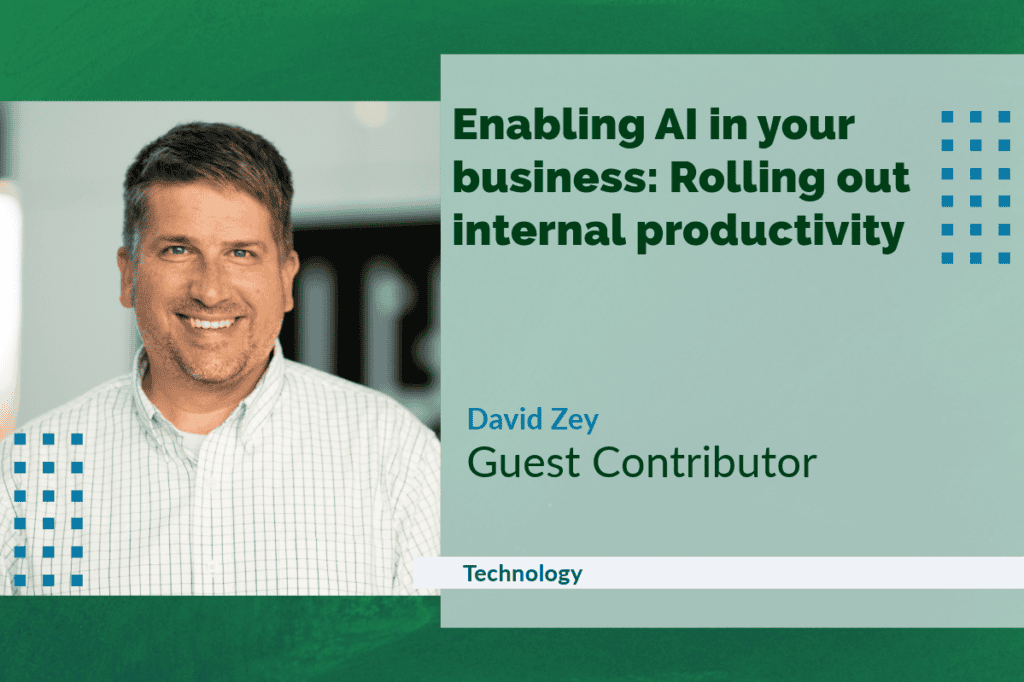
July 15, 2024
In high school, I applied for a full scholarship to a local liberal arts college.
As part of the competition, I was given a sealed essay prompt with one hour to complete it.
The prompt asked whether the atomic technology ending World War II was inherently good or evil.
As a high schooler, that was a challenging topic for an impromptu essay.
I argued that technology is morally neutral because it is inert (a tool) – it is the intent of use by humanity that imbues technology with morality.
With hindsight, I would have added that technology employed in the absence of human intent is not morally neutral either, as it often leads to unintended consequences.
Avoiding negligent use of technology through intentional stewardship is essential for leveraging AI in business teams.
This approach can help secure responsible outcomes and increase the efficacy of AI solutions within organizations.
Organizational stewardship for AI
There are different forms of stewardship within organizations, but two are particularly relevant to AI:
Data stewardship is the responsibility of all organization members to organize, store and maintain information assets in approved physical and digital locations.
These assets should be accessible to authorized personnel based on their organizational roles and restricted from unauthorized access.
The relevance of data stewardship to AI is that it relies on high-quality, well-organized data for effective training and operation.
It can be trained on organizational data sets to catalog business processes and information for inquiry.
Ensuring data security, especially in transit, is crucial to maintaining network security and preventing unauthorized access – which needs to be factored into your organization’s data stewardship practices.
Intent stewardship involves the responsibility of all organization members to guide their use of technology, tools and power to ensure ethical, responsible and purposeful outcomes on behalf of the organization.
It emphasizes deliberate and conscious intent in decision-making to avoid negligence and unintended consequences.
The relevance of intent stewardship to AI is that it empowers teams to function at a higher level of scale and quality, but unattended use can lead to unintended consequences.
AI does not inherently validate source data – its responses are based on training data, patterns and repetition found in the data, human reinforcement and post-learning algorithms.
Therefore, users must evaluate AI outputs to ensure they align with ethical and responsible use.
A practical assessment of knowledge loss in organizations
Because AI is a form of automated cognition, there is a risk of losing understanding over time in how certain thought-based processes work within the organization.
This can lead to the atrophy of both individual and collective organizational knowledge.
Though concerns about employee knowledge loss through AI are legitimate, AI also offers an upside – it can capture and preserve organizational knowledge, thereby transcending the succession and loss of trained talent.
Since COVID-19, significant lateral, vertical and geographical movement of professionals has disrupted the normal succession of roles and knowledge within organizations.
The gig economy has empowered professionals to curate their marketability and opportunities, leading to increased worker transience.
This trend may correct over time, but professionals have gained leverage, the economy has tightened and organizational churn has increased.
This strain on the training process is problematic because it is important but costly to accurately convey processes, documents and role knowledge to new employees.
Additionally, it is challenging to capture the knowledge that evolves with an employee before their exit interview.
This attrition of knowledge can be mitigated with custom AI models trained on trusted organizational data.
As we discuss productivity gains within business teams, it’s essential to consider the benefits of AI tools that specifically understand your organization.
Tools like Microsoft Copilot can account for your existing organizational data (underscoring the need for appropriate data stewardship).
Discussing productivity tool options
There are several classes of AI tools on the market one may consider in light of data and intent stewardship, including:
General LLMs
ChatGPT, Azure Cognitive Service and Vertex AI are well-known players.
These offer extensive capabilities as general models that can learn from user conversations and integrate into cloud environments.
However, they do not prioritize organizational data and security as discussed, posing additional concerns about company information in transit and at rest.
Integrated productivity AI
Microsoft Copilot and Gemini for Google Workspace integrate information from commonly used productivity tools like Word, Google Docs, Excel, Google Sheets, Teams and Google Meet.
They provide contextualized responses based on role-based access to user data.
Integrated ERP/CRM AI
Salesforce Einstein, IBM Watson Assistant and Zoho Zia understand the specific structured data of ERP or CRM systems, capturing different slices of organizational data.
They support narrower use cases centered around specific business processes and have role-based security constraints.
These niche AI tools also limit in-transit data security concerns within the ERP or CRM system they are designed for.
Open-source general LLMs
GPT-Neo, GPT-J and LLaMA are smaller, open-source models compared to GPT-3 and GPT-4 and can be deployed on-premises, offering more control over data security and data sets.
These models can effectively capture the essence of an organization’s knowledge and processes, becoming valuable corporate assets of Intellectual Property (IP) for certain types of companies.
An organization may acquire multiple LLMs or AI models to support different user groups.
Each AI tool will have its own IT vetting, hardening, business training and stewardship training concerns.
General and integrated AIs receive vendor support from providers like Microsoft, Google, Salesforce, IBM and Zoho.
Open-source LLMs come with varying licenses (e.g., MIT, Apache 2.0, non-commercial) and rely on community support or third-party service partners.
Applying AI for productivity
There are many ways to think about productivity gains with AI, and it’s easy to list general applications like content generation, email writing and meeting summaries.
However, applying a value-effort matrix adds more structure and clarity when training teams. Traditionally in a value-effort matrix, quick wins (high value, low effort) get the highest priority, while thankless tasks (low value, high effort) get the lowest priority.
AI can transform some high-effort tasks into low-effort ones, thus reprioritizing the matrix and often improving quality.
Using this matrix with an AI perspective, here are some AI applications to consider:
Quick wins (high value, low effort)
- Data insights summary: Summarizing key insights from sales data in Excel
- Email management: Drafting follow-up emails for sales leads
- Document drafting: Creating standard documents or templates in Word
- PDF summarization: Summarizing key points from PDF documents for quick review
Major projects (high value, high effort shift to less effort)
- Project management: Assisting with creating and managing project plans using an existing company work breakdown structure (WBS)
- Customer support: AI chatbots can handle routine inquiries, significantly lowering the effort needed for customer service tasks
- Strategic planning: Developing detailed strategic plans or business reports in Word
- Contract evaluation: Analyzing contracts and business terms for key points and potential issues
Fill-ins (low value, low effort)
- Basic data analysis: Performing simple data analysis tasks in Excel
- Simple reports: Generating basic reports from predefined templates in Excel
- Document formatting: Applying standard formatting to documents in Word
- Meeting agendas: Creating agendas for regular team meetings
Thankless tasks (low value, high effort shift to less effort)
- Complex formatting: Applying detailed formatting to extensive documents in Word
- Data entry: Entering data from various sources into a single Excel sheet
- Meeting minutes: Transcribing lengthy meeting recordings into detailed minutes in Word
- Inventory optimization: Analyzing inventory data to recommend optimal stock levels
Using this structured approach, businesses can effectively harness AI to boost productivity by prioritizing tasks offering the greatest return on effort.
AI can transform traditionally high-effort tasks into low-effort ones, shifting priorities and enabling teams to focus on higher-value activities.
This can enhance productivity but also help promote intentional stewardship of technology.
By creating a repeatable framework for training teams and managing data, businesses ensure their use of AI is responsible and innovative, driving sustained productivity and growth.