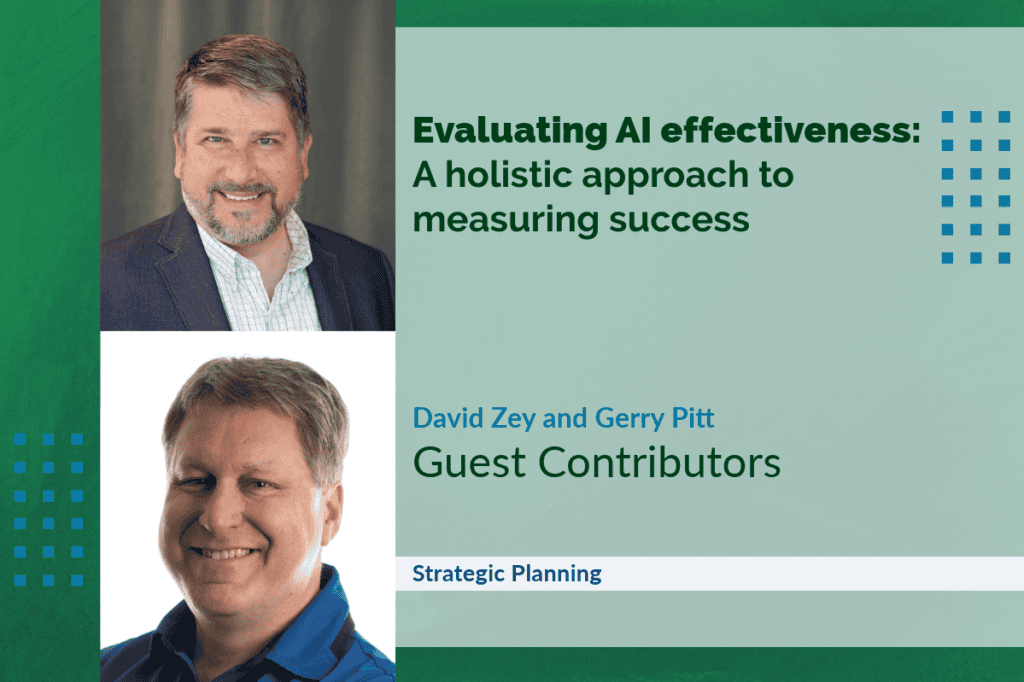
May 5, 2025
The rapid adoption of AI technologies across multiple industries has brought about significant changes in how businesses are looking at their operations.
Though AI has the potential to streamline processes, enhance productivity and drive innovation, many organizations face challenges in measuring the success of their AI investments.
And while the benefits of AI are widely recognized, understanding how to measure its impact can be complex.
This column offers a straightforward look at how to get real business value from AI, starting with practical ways to think about ROI, spot early wins and track progress.
By adopting a structured approach, businesses can ensure their AI initiatives deliver real value and drive long-term success.
The importance of strategic planning
Avoiding the pitfalls of rushing AI projects
One of the key challenges organizations face is the pressure to quickly demonstrate ROI from AI projects.
When AI adoption is driven more by urgency than intentional strategy, organizations risk scaling back too soon.
Many organizations expect quick returns on their AI investments, but this urgency can lead to shallow implementations, abandoned projects and missed opportunities for lasting transformation.
The pressure to deliver immediate results often overlooks the exploratory nature of AI innovation, a process not unlike the early days of “data mining,” where the real value came not from instant success, but from digging deep, testing assumptions and unearthing hidden insights over time.
To avoid these pitfalls, organizations should set realistic expectations and establish key metrics to measure the value of AI deployments.
Targeted AI projects that address specific organizational problems tend to show more promise than generic, general-purpose applications.
For example, using AI in manufacturing for predictive maintenance or quality control can provide measurable gains in productivity and cost savings.
The role of patience in AI implementation
Expecting immediate returns from AI investments can be unrealistic.
AI projects often require time to mature and demonstrate their full potential.
For instance, an appointment scheduling chatbot may initially be 75% accurate.
Over time, with continuous feedback and refinement, its accuracy can improve to the desired 85-90%.
This gradual improvement emphasizes the importance of patience and iterative development in AI projects.
Organizations should also recognize that AI’s ROI will come in incremental steps rather than all at once.
This step-by-step approach allows for continuous improvement and adaptation, helping to ensure that AI tools are effectively integrated into business processes.
Early adoption: Speed over perfection
In the early stages of AI adoption, businesses should prioritize speed and learning over polish and completeness.
Applying the logic of the iron triangle, where cost, quality and speed compete, AI ROI often comes from increasing speed while accepting moderate quality.
This tradeoff isn’t a flaw – it’s a feature.
By automating repeatable tasks or assisting decision-making, organizations can reduce effort, shorten turnaround time and uncover areas for deeper improvement.
Even if quality remains moderate compared to existing high-touch or manual processes, the gains in efficiency and insight justify the investment.
Year one ROI, figuratively speaking, is about creating traction, not perfection and setting the stage for scalable, higher-quality outcomes ahead.
Measuring AI’s success: A holistic approach
Four essential categories
- Compliance
- Quality
- Employee experience
- Business impact
Due to the absence of a standardized method, many organizations are unsure if their AI investments are truly valuable.
Some prioritize workforce productivity, while others focus on revenue growth or innovation. However, relying on isolated metrics can lead to an incomplete understanding, making it difficult to decide which AI tools to expand, refine or discontinue.
This framework categorizes top AI metrics into practical groups, explaining what each metric reveals and how it fits into the broader ROI picture.
Compliance
Compliance is often overlooked as an ROI measure because its value is hard to quantify.
Only a small percentage of business leaders consider it a measure of AI success.
However, compliance is a crucial initial checkpoint for evaluating any AI tool or initiative.
Before an AI tool can provide value, it must meet security, privacy and regulatory standards.
Common measures:
- Data encryption, security certifications (e.g., SOC 2, PCI DSS, ISO 27001)
- Regulatory compliance (e.g., GDPR, HIPAA, SOX)
- Access controls and user permissions
- Security Incident response and historical breach data
Effective measurement techniques:
- Regular audits
- Compliance checklists
- Incident management systems
- Policy review and updates
Compliance alone does not indicate effectiveness or usability.
A tool can be secure but still fail in quality, adoption or impact.
Ensuring that AI tools meet compliance standards is the first step in mitigating risks such as data breaches and regulatory fines, which can undermine trust and expose the business to unnecessary risks.
Quality
The value of an AI tool is determined by the quality of its output.
Whether generating content, analyzing data or automating processes, AI must consistently produce accurate, relevant and trustworthy results to drive real business impact.
Quality is a critical indicator of AI success, with many business leaders using these metrics to evaluate performance.
Concerns about AI producing outdated, inaccurate or misleading content are common, making quality a crucial point in assessing AI’s true impact.
Common measures:
- Error rates (e.g., grammatical, factual or analytical mistakes)
- Communication metrics (e.g., clarity, readability, effectiveness)
- A/B testing results for AI vs. human performance
Effective measurement techniques:
- Statistical process control (SPC)
- Sampling
- Inspection
- Customer feedback
Quality alone does not measure efficiency, adoption or business impact.
A highly accurate tool that is slow, difficult to use or poorly integrated may still fail to deliver ROI.
Therefore, it can be essential to consider quality alongside other metrics to ensure that AI tools are both effective and efficient.
Employee experience
AI’s ROI metrics, such as productivity, innovation and efficiency, are often realized at the individual employee or user level. However, AI’s true value emerges when usage scales beyond individuals to entire teams and functions. When AI is integrated into daily workflows across departments, its impact multiplies, enhancing collaborative efficiency, knowledge-sharing and organization-wide improvements. Utilization is a leading indicator of AI ROI and a measure business leaders can use when considering scaling a tool or initiative. Despite significant investments in AI tools to foster innovation and improve productivity, less than one-third of business leaders measure these metrics, potentially overlooking AI’s true impact on workforce efficiency and creativity.
Common measures:
- Weekly hours saved
- Increased output per employee
- Task automation rates
- AI response confidence score
Effective measurement techniques:
- Employee surveys
- Focus groups
- Employee Net Promoter Score (eNPS)
- Exit interviews
Productivity gains do not necessarily mean better outcomes because faster work is not always more effective.
This metric should be assessed alongside quality and impact to ensure AI is driving both effectiveness and efficiency.
By embedding AI into daily workflows, organizations can achieve significant improvements in collaborative efficiency and knowledge sharing.
Business impact
AI’s success must be measured by tangible business outcomes.
Whether through cost savings, increased revenue, stronger customer relationships or greater operational speed, AI should influence the bottom line.
Despite recognizing AI’s potential impact on these metrics, most business leaders do not have tracking measures in place to prove it.
By monitoring AI’s influence on key business metrics, organizations can determine whether their investments are truly driving bottom-line value.
Common measures:
- Reduction in operational costs (e.g., labor, infrastructure)
- Reduction in number of open-worker requests (current workforce scaling)
- Streamlining the technology infrastructure
- Standard operating procedures streamlined or automated
Effective measurement techniques:
- Key performance indicators (KPIs)
- Balanced scorecard
- Cost-benefit analysis (CBA)
- Benchmarking
Cost reduction alone does not account for the strategic value AI may bring.
A tool that cuts costs but diminishes quality or experience may not deliver long-term benefits.
Therefore, it is crucial to consider the broader impact of AI on business operations and strategic goals.
Conclusion
No single metric fully captures AI’s return on investment, as its impact spans across teams, workflows, strategic decision-making and customer experience.
Without clear tracking mechanisms, organizations risk investing in AI tools that fail to deliver meaningful results or, worse, overlooking AI solutions that could be transformative.
The ROI of AI is determined by a combination of factors across compliance, quality, employee experience and productivity and business impact.
A refined, flexible approach to measurement allows business leaders to assess AI’s effectiveness holistically, ensuring that investments align with strategic goals.
For organizations seeking to implement this framework, adopting a structured, data-driven approach will be crucial.
AI is no longer a future investment; it’s reshaping the way businesses operate today.
However, without a clear way to measure success, even the most advanced AI tools risk becoming underutilized or misaligned with business goals.
Business leaders who take a methodical approach to AI integration will be the ones who maximize its potential.
The journey to achieving meaningful ROI from AI investments requires careful planning, patience and a comprehensive approach to measurement.
By focusing on compliance, quality, employee experience and business impact, organizations can ensure that their AI initiatives deliver real value and drive long-term success.